Lancet Digital Health - 01 July 2022
Combining the strengths of radiologists and AI for breast cancer screening: a retrospective analysis
- Christian Leibig et al.
Original Article: https://www.thelancet.com/journals/landig/article/PIIS2589-7500(22)00070-X/fulltext
Summary:
- The largest retrospective study of its kind into the use of artificial intelligence as a tool to assist radiologists with breast cancer screening has demonstrated that the technology can significantly improve screening accuracy - leading to more accurate diagnoses - without sacrificing mandatory high safety standards.
- The study, published in The Lancet Digital Health and commissioned by deeptech firm Vara, evaluated the performance of an AI-based approach using mammograms from more than 100,000 women in Germany, including more than 4,400 with identified cancers. Radiologists and researchers found that when AI is implemented along the screening pathway in a way that complements, rather than replaces human radiologists both sensitivity and specificity of radiologists improved significantly. Different algorithm configurations were evaluated, with some demonstrating that over 70% of the workload could be automatically triaged by the AI.
- The success of this approach, known as decision referral, suggests that the most effective way to incorporate AI technology into clinical practice is as a tool used by radiologists, helping them to make better decisions and better manage the administrative burden of their task.
- Vara’s AI technology optimises performance of both normal triaging and cancer detection. Normal mammograms are triaged and their respective structured reports are automatically generated, while mammograms assessed by the reader receive additional post-hoc AI cancer detection support when needed.
- This key feature of decision referral, the safety net, is triggered only when AI disagrees with the reader, flagging potentially missed cancers. In direct contrast to traditional computer aided detection (CAD) systems which have struggled with increased false positives, Vara’s decision referral approach may prove to be the optimal use of AI to find low-prevalence cancers without biasing the reader negatively.
- The complementary processes were shown to outperform both the average (unaided) radiologist and the use of AI in stand-alone mode – that is, when the AI was working independently. In some cases, the decision referral approach could improve radiologist sensitivity by up to 7.2 percentage points.
- Existing approaches to adopting AI in screening have focused on using the technology to replace radiologists by allowing the technology to interpret mammograms on their behalf. These approaches, while presented as the most promising route for the technology to be implemented into clinical settings, have been repeatedly criticised.
- Vara's decision referral specifically combines the strengths of both radiologists and AI by taking a case-by-case approach to assessing the mammograms. Where the AI is not confident enough, it refers the decision to radiologists. This also creates potential to save workload.
- A total of 104,518 mammograms from between 2007 and 2020, including 4,463 screen-detected cancers from eight screening units participating in Germany’s national mammography screening program were used to evaluate the algorithm’s performance. Mammograms from six screening units were used as an internal test set, while data from two further screening units were used as an external test set.
- The authors compared the performance of two distinct screening approaches for AI - a stand-alone AI approach, and the AI decision referral approach. The sensitivity and specificity of each approach was evaluated using the two sets, and compared to the average unaided radiologists' real-world performance on the same mammograms.
- The unaided radiologists achieved higher sensitivity and specificity across both sets compared to the stand-alone AI.
- However, when using the decision referral approach, these scores surpassed those of the radiologists alone. In one example configuration, the approach improved radiologist sensitivity by 4.0 and 2.6 percentage points on the internal and external sets, respectively. With high accuracy, this approach allowed AI to independently perform normal triaging and cancer detection leading to over 60% of screening mammograms automatically triaged at this configuration.
Authors:
Christian Leibig, PhD*1; Moritz Brehmer, MD*1,2; Stefan Bunk, MSc1; Danalyn Byng, MSc1; Katja Pinker MD†,3,4; Lale Umutlu, MD†,2
1Vara, Berlin, Germany. 2Department of Diagnostic and Interventional Radiology and Neuroradiology, University-Hospital Essen, Germany. 3Department of Radiology, Breast Imaging Service, Memorial Sloan Kettering Cancer Center, New York, NY, USA. 4Department of Biomedical Imaging and Image-guided Therapy Division of Molecular and Gender Imaging, Medical University of Vienna, Vienna, Austria.
*Joint first authors, †Joint last authors
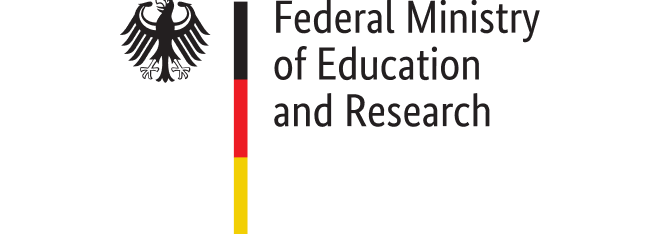
The project on which these publications are based was funded by the Federal Ministry of Education and Research under grant number 01QE2002. The responsibility for the content of this publication lies with the author.